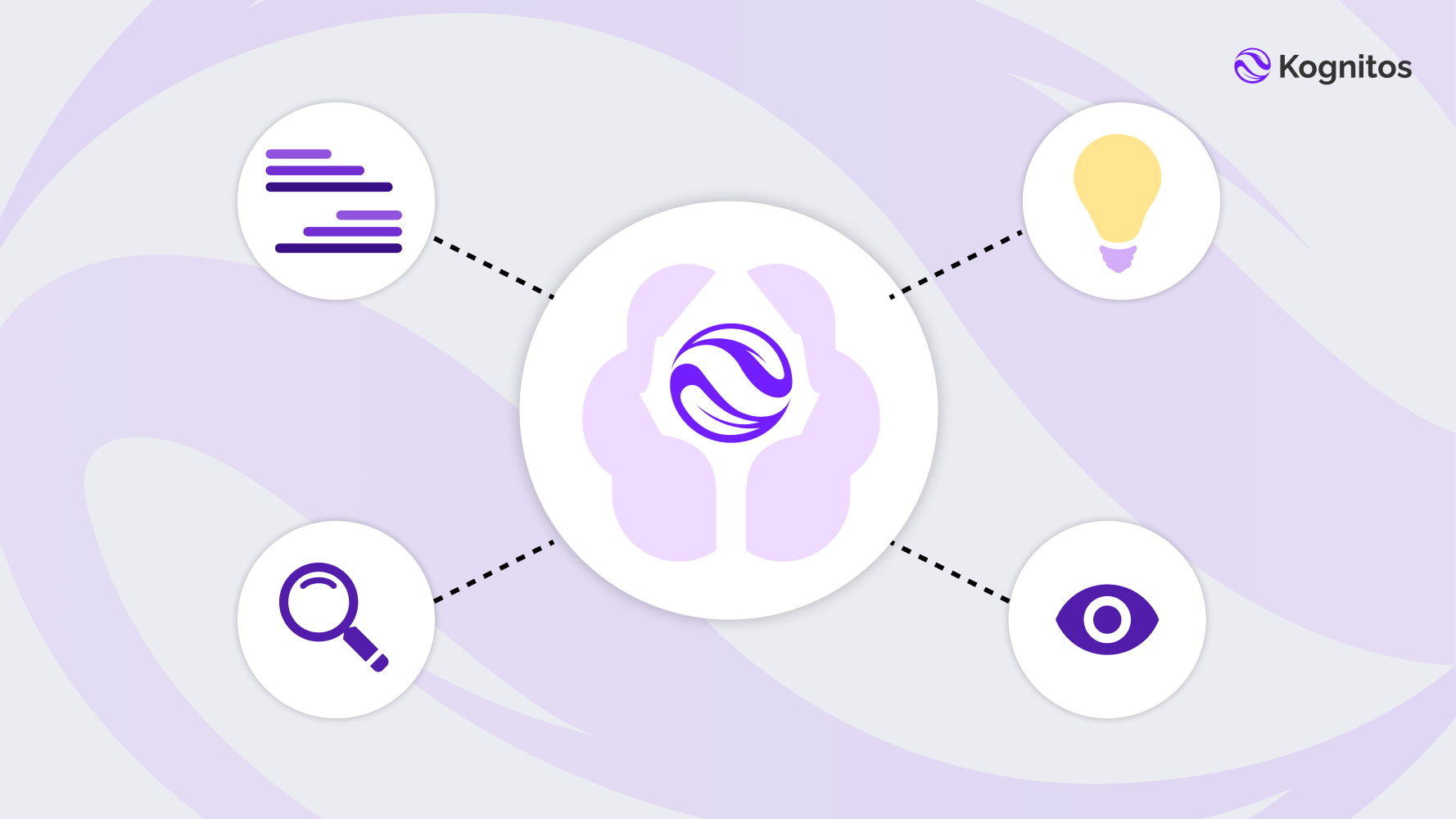
Artificial intelligence (AI) has rapidly gone from an abstract concept in computer science or a science fiction trope to a real-world technology impacting virtually every industry and job role. Generative AI takes it a step further, as automation evolves to new levels of sophistication and creativity—although not without its flaws (see: hallucinations).
Machines are now able to analyze and interpret data, along with creating content, designs, and even code. In this blog, we’ll explore the origins of AI, what makes generative AI unique, and its growing role in business process automation.
Introduction to AI: A Brief History
AI has its roots in the mid-20th century. Alan Turing did the most foundational work simply by asking if machines can think. The field of AI was pioneered by Alan Turing, John McCarthy, and Marvin Minsky, relying on rule-based systems to automate basic tasks like playing chess or solving math problems
The 1956 Dartmouth Workshop, often considered the birth of AI as a formal academic discipline, explored how machines could simulate human intelligence through logic, reasoning, and symbolic computation.
For decades, AI evolved slowly, hindered by limited computational power and data availability. But in the late 1990s and early 2000s as computing power pricing plummeted, AI research exploded. Since then, we’ve seen exponential growth and acceptance of AI, along with the rise of neural networks, deep learning, and more complex tasks like image recognition, natural language processing, and autonomous driving.
Generative AI and agentic AI are at the forefront of a new era. But what exactly differentiates generative AI from traditional AI?
What Makes AI Generative?
Simply put, generative AI creates something new. It goes beyond analyzing data inputs to generate new content, mimicking human creativity. Traditional AI models focus on identifying patterns or classifying data. In contrast, generative AI is capable of producing, for example, a new image, piece of music, or text passage based on patterns it has learned from previous datasets.
Generative AI relies heavily on deep learning techniques, particularly models like:
- Generative Adversarial Networks (GANs) consist of two neural networks. The generator creates new data, while the discriminator evaluates how realistic the generated data is. As the model learns, its outputs become more and more realistic.
- Transformers, like OpenAI’s GPT models, understand context at a deep level. They use vast amounts of data inputs to generate sequences—often words or code.
- Variational Autoencoders (VAEs) are used primarily in image generation. They encode data into a latent space, and then decoding it back into a form.
While no generative AI can create human output, these technologies mimic it to an impressive degree.
Use Cases for Generative AI in Process Automation
Generative AI has become a game-changer for process automation. Businesses increasingly look to AI to streamline tasks, embellish creativity, and improve decision making. Let’s explore some of the top use cases:
- Demand forecasting: Automate the collection and analysis of historical sales data, market trends, and other relevant factors to generate accurate demand forecasts.
- Inventory tracking and reconciliation: Continuously monitor inventory levels, automate reorder processes, and reconciles physical counts with system data.
- CRM data management: Ensure data accuracy and consistency in CRM systems by automating data entry, updates, and cleansing processes.
- Sales order processing: Streamline the sales order process by automating order entry, validation, and fulfillment.
- Production reporting: Automate the collection and consolidation of production data from various sources. Generate real-time production reports, calculate KPIs, and more.
- Production scheduling: Analyze demand forecasts, inventory levels, and resource availability to generate production schedules, allocate resources, and adjust plans.
- Compliance monitoring and auditing: Monitor business processes for compliance with internal policies and external regulations, generate alerts for violations, automate audit processes, and produce compliance reports.
- Software installations and updates: Deploy software updates and patches across multiple systems, schedule installations, verify successful updates, and generate reports on the status of software across the organization.
- Time and attendance tracking: Automatically collect and process employee work hours, flag discrepancies, calculate overtime, and generate reports for managers, ensuring accurate payroll and compliance.
- Customer feedback analysis: Categorize feedback, identify trends, generate reports, and trigger alerts for urgent issues, enabling proactive customer service.
The Future of Generative AI in Automation
Generative AI has already had an undeniable impact on business process automation. We expect to see systems become more sophisticated and seamlessly integrated with human workflows.
In the future, we might even see fully autonomous creative teams, AI-driven innovation in scientific research, or personalized education tools that adapt content to individual learning styles. For now, however, we’d be remiss to not acknowledge the ethical challenges that come with generative AI, including bias and discrimination, privacy and security, and misinformation.
Generative AI represents a significant leap in the evolution of artificial intelligence. It moves beyond analysis, to innovation. As generative AI technologies continue to advance, we will undoubtedly witness groundbreaking applications across industries.
How Kognitos Leverages Generative AI
Kognitos harnesses the power of generative AI to revolutionize business process automation. At the heart of its architecture are two crucial Large Language Model (LLM) layers that enable the platform to understand, create, and manage complex automations with unprecedented ease and efficiency.
The first LLM layer, known as the Business Logic Model, serves as the cornerstone of Kognitos’ ability to translate natural language instructions into actionable automation steps. This sophisticated model interprets user input, breaking down complex process descriptions into clear, structured logic. The Business Logic Model can understand the nuances and intent behind user instructions, even when they’re expressed in everyday business language. This allows Kognitos to bridge the gap between human thought processes and machine-executable actions, effectively democratizing the creation of automation workflows.
Complementing this is the Exception Handling Model, a second LLM layer that addresses one of the most challenging aspects of process automation: managing unexpected issues. When an automation encounters a problem or an unforeseen scenario, this AI layer springs into action. It analyzes the situation, interprets the error in context, and then presents the issue to users in plain, conversational language. This approach allows business users, regardless of their technical expertise, to comprehend and address problems quickly simply by answering questions and providing guidance. The process is paused while the user provides input or guidance, ensuring that automations remain under human control even as they handle complex scenarios autonomously.
Together, these LLM layers sets Kognitos apart in the automation landscape. This use of generative AI makes Kognitos particularly effective for document-heavy processes that often require nuanced decision-making. While other technologies are rushing to use generative AI in process creation, they aren’t equipped to manage the challenges of hallucination and edge cases in the same ways that Kognitos boasts today.
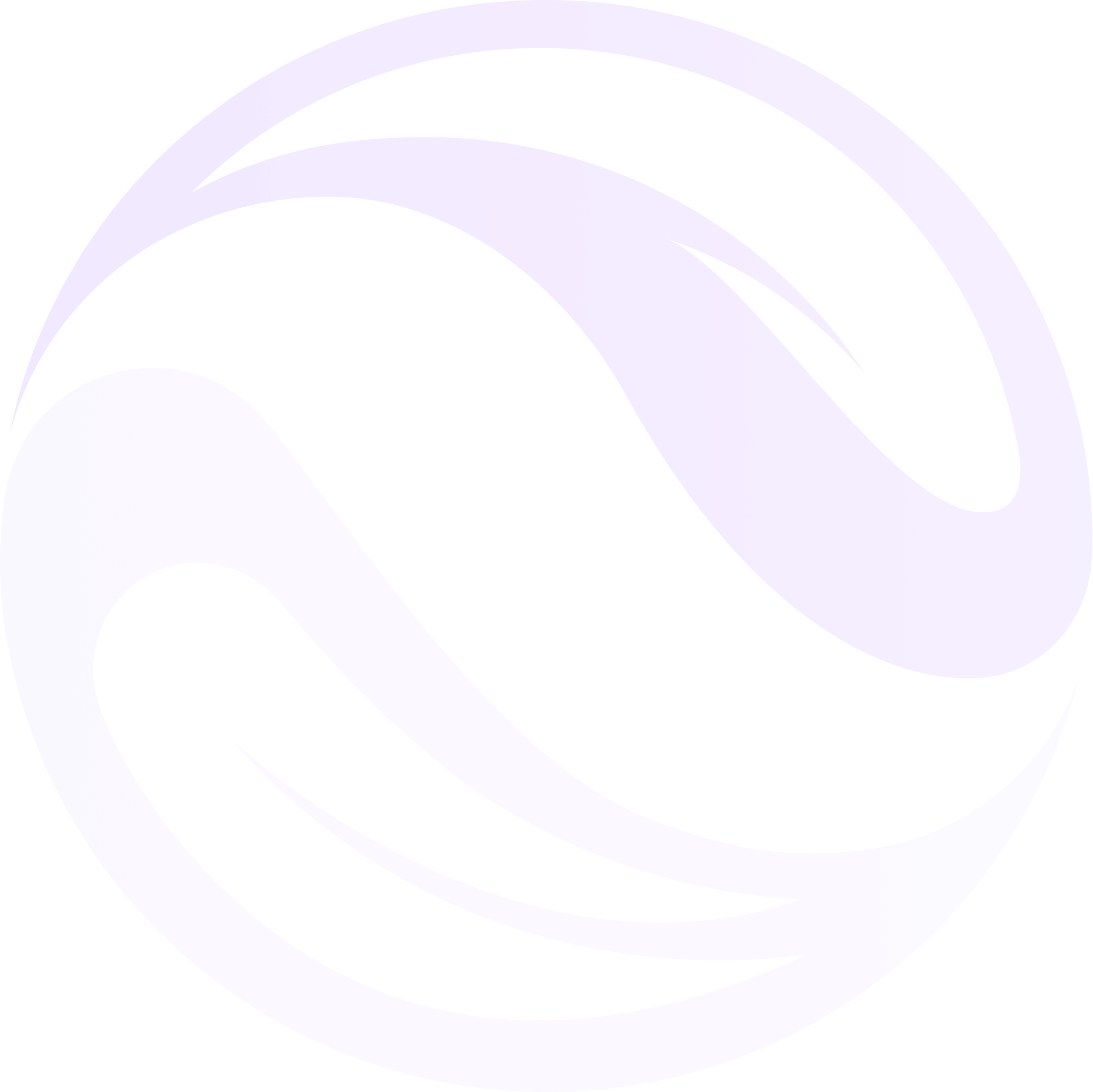
Discover the Power of Kognitos
Our clients achieved:
- 75%manual data entry eliminated
- 30 hourssaved on invoicing per week
- 2 millionreceipts analyzed per year