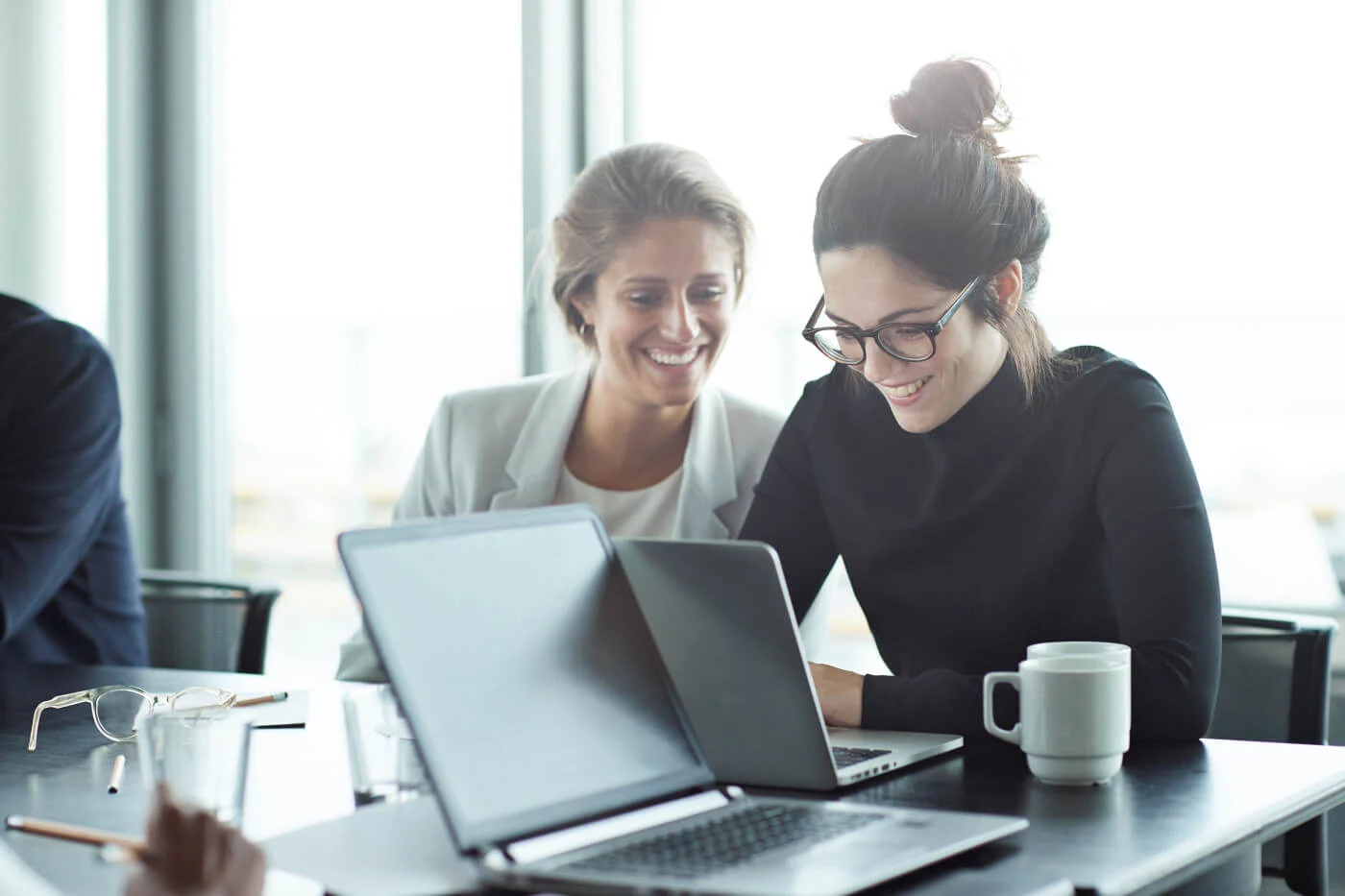
Many businesses leverage OCR + RPA to save time and reduce errors associated with manual data entry. For example, a manufacturing company that processes thousands of invoices per month that are highly standardized and do not vary often, can use OCR technology to extract invoice data automatically. Today some forms of OCR are becoming available to business users through no-code templates and pre-trained models, but most require some developer expertise. These technologies are a good first step, but unfortunately only cover a small portion of the total documents used by businesses.
Limitations of Current Approaches
Despite its strengths, OCR and RPA both have limitations that restrict the ability to process complex documents. OCR technology has limited ability to recognize unstructured text like handwriting, non-standard fonts, and poor image quality. Furthermore, OCR technology has limited accuracy rates in understanding the context and extracting information from the wider business process. It is particularly challenging to extract information from complex documents that include tables, graphs, and other visual elements. OCR technology also struggles to process documents with incomplete information, and it is difficult to catch and handle errors using OCR technology alone.
RPA functions much in a “Bad Data In, Bad Data Out” style. When fed bad or incomplete data by OCR, RPA bots frequently break and are described as “Brittle”. Additionally, RPA is best when used for processes that do not have lots of exceptions. Complexity or variations in documents can create exceptions that cause RPA bots to break.
OCR + RPA is a great tool for standardized processes, but struggle with different document types. These limitations of OCR technology and other traditional approaches to document processing are holding back businesses in today’s fast-paced market. Businesses need accurate and efficient document processing to make informed decisions, streamline workflows, and maintain a competitive edge. Incomplete data, inconsistent data, and errors in document processing can cause businesses to lose money and damage their reputation.
How can Generative AI overcome the above limitations?
Generative AI like ChatGPT, or GPT4 is a game-changer for document processing. It uses advanced deep learning algorithms to analyze large volumes of data and identify patterns, making it highly accurate and efficient in document processing. Generative AI can learn from diverse examples and adapt to new data inputs over time, making it highly effective in processing complex documents with tables, graphs, and other visual elements. It can also recognize and understand the context of the wider business process, making it highly effective in handling incomplete or inconsistent data.
For example, a hospital can use Generative AI to process medical charts, which contain complex data structures such as tables, diagrams, and graphs. By using Generative AI, the hospital can extract critical information from the medical charts, such as patient diagnoses, medications, and treatments, with a high degree of accuracy and speed.
In another example, Kognitos worked with an international conglomerate that needed to match payments with invoices from different subsidiaries across the globe. The complexity of the process and the variety of documents and languages necessitated a tool that could understand context, and financial professionals in a shared services center to directly control the automation, not developers. By combining Generative AI with OCR in a Generative AI Automation platform, the conglomerate could greatly reduce the number of people manually processing data with high degrees of accuracy.
Generative AI can also help businesses handle cases when documents have incomplete data or when OCR has extracted incorrect information. It can recognize the context of the wider business process, understand the relationships between different pieces of information, and use this information to extract the correct data. For example, an insurance company can use it to process claims that contain incomplete or inconsistent data. The claims processor rather than a developer, is in control, and can teach Generative AI Automation how to handle situations with incomplete data, and how to find that data in future documents. An example of how a business user can teach Generative AI automation how to handle a document with a simple command can be found here: Generative AI + OCR
In conclusion, traditional approaches to document processing such as manual data entry and OCR technology helped take the first step, but have significant limitations that can hold back businesses. Generative AI is a game-changer for document processing, providing accurate and efficient processing of complex documents. By implementing Generative AI Automation for their document processing needs, businesses can streamline their workflows, decrease errors, and reduce the need for developers, greatly lowering the cost of automation and the ROI of projects. This gives companies a competitive edge in today’s fast-paced market. The time to adopt Generative AI for document processing is now.
Want to Unlock the Power of Generative AI for Your Business Today
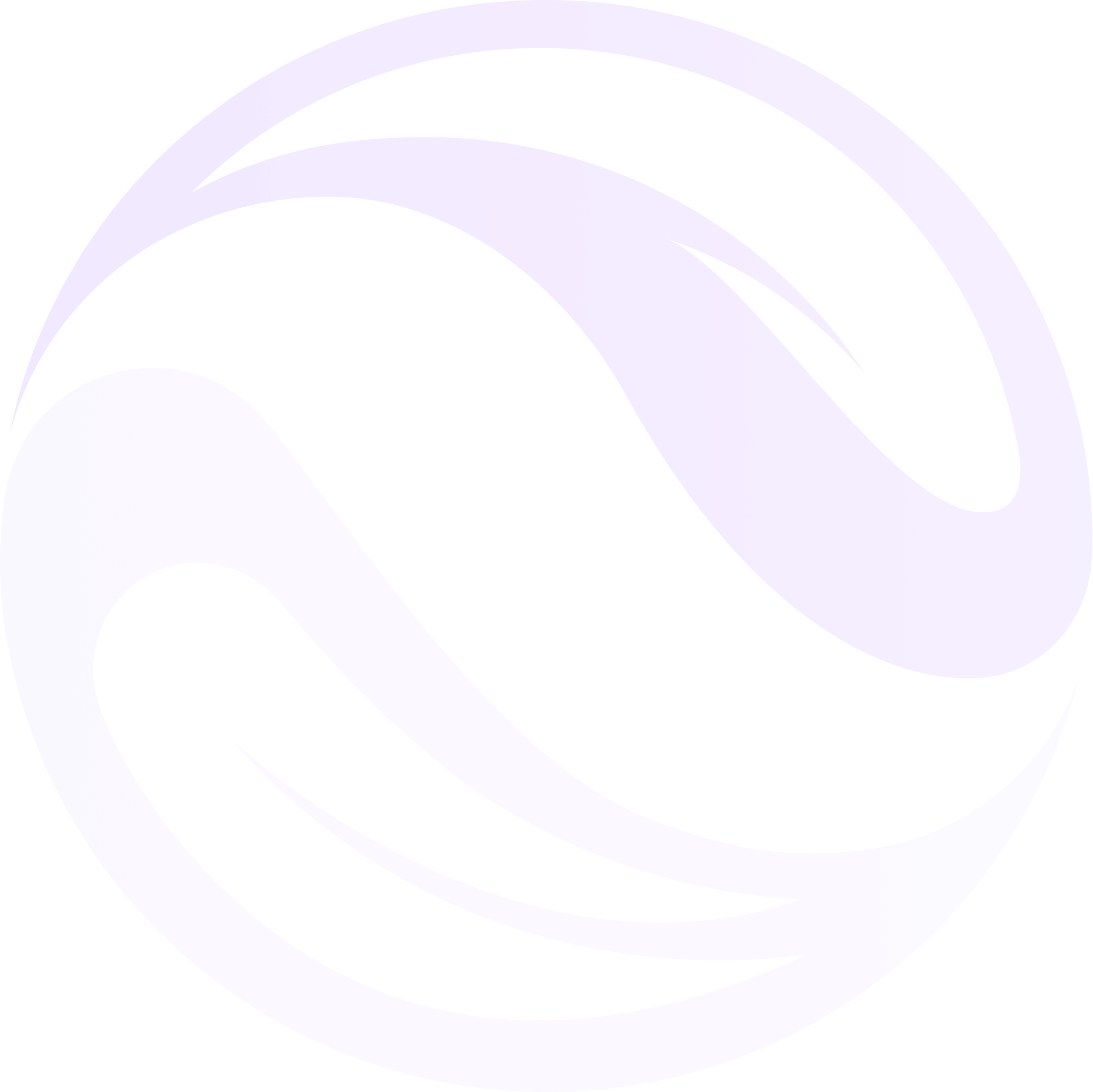
Discover the Power of Kognitos
Our clients achieved:
- 75%manual data entry eliminated
- 30 hourssaved on invoicing per week
- 2 millionreceipts analyzed per year